To reduce wildlife deaths caused by fences, scientists are turning to AI
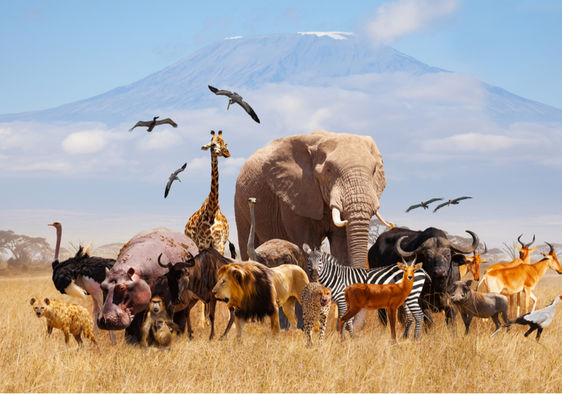
Software trained to identify fences from aerial images could help wildlife managers prevent pronghorn from getting stuck and starving
As many as 1 million kilometers of fence may crisscross the western United States, enough to stretch to the Moon and back. Erected over the past century, largely to contain livestock, the barbed and woven wire fences also hinder wildlife such as deer, elk, and pronghorn from migrating to find food or escape deep snow. Conservationists are trying to remove or replace some old fences—but that means finding them in the vast expanses of the West. Two researchers turned to artificial intelligence (AI) for help.
Wenjing Xu, a postdoctoral researcher at the Senckenberg Biodiversity and Climate Research Centre, and Zhongqi Miao, an applied research scientist with the Microsoft AI for Good Lab, trained a computer to identify fences in aerial images taken from planes flying over southwestern Wyoming, an area that’s important for migratory pronghorn and mule deer. Checking the fencing estimates with data from on-the-ground surveys, they found the system could accurately identify about 70% of the fences, they reported last week at the Ecological Society of America conference in Long Beach, California. The duo plans to expand its methods to use satellite images, which could open the door to identifying fences elsewhere in the world.
“Fences play a large role in how animals move and the health of the environment, and currently it’s really hard to get good data about what’s going on with them,” says Ben Koger, an ecologist and computer scientist at the University of Wyoming who also uses aerial images in his research but was not involved in the project. Miao and Xu’s team has “taken a good step towards doing something about that.”
Fences have long been a problem for wildlife across the West that migrate to find the best habitat, as one recent study underscored. Western EcoSystems Technology biologist Hall Sawyer radio collared 45 pronghorn to track their movements in a stretch of high plains in Wyoming called the Red Desert. In the winter of 2023, after months of deep, wet snow, strong winds, and cold temperatures, half of the collared pronghorn died—along with thousands more from the overall herd. Their collar data showed the animals had tried to escape the harsh winter conditions—only to bump into fences and an interstate highway. One animal had wandered well over 400 kilometers but never managed to get farther than 50 kilometers from where it started, Sawyer says.
In some areas, wildlife managers and conservation groups have begun to take out fences or replace them with wildlife-friendly fencing that’s easier for species such as pronghorn to crawl under and elk to hop over. A 2019 paper that looked at the impact of fence removals in Alberta, Saskatchewan, and Montana found that because pronghorn strongly avoided fences, removing the barriers could increase access to high-quality forage for the animals by up to 38% across both public and private land.
It can be difficult to systematically remove or replace fences, however, when you don’t know where they are. “Public agencies have records in some places and don’t in others,” says Xu, who worked to map more than 7000 kilometers of fence through on-the-ground surveys in southwestern Wyoming for her Ph.D. at the University of California, Berkeley.
To try to create a better inventory, she teamed up with Miao to develop a method for identifying large tracts of fences remotely. So far, they have focused on photos taken from planes that have a resolution down to 60 centimeters per pixel. Miao trained the deep-learning model to look for fences in each pixel using the on-the-ground data from Xu’s work in Wyoming. Miao calls the results “really promising” but acknowledges early limitations, including cases when the model has mistaken roads for fences. He and Xu are now looking for collaborators with whom they could collect higher resolution images and train the model on them, to make it more discriminating.